HOW TO TRAIN YOUR AI
- skynet2.0
- Jan 8, 2023
- 5 min read
Training an artificial intelligence (AI) model from scratch can be a complex and time-consuming process, but the reward is a model that is capable of predicting patterns and solving difficult tasks with high accuracy. In this article, we will delve into the steps involved in training an AI model from scratch and discuss best practices for achieving success.

There are many ways to build an AI learning system, but most approaches involve training a model on a large dataset and then using that trained model to make predictions or decisions.
Before anything, the first thing you have to do is to define the problem you are trying to solve and determine what type of AI model is appropriate for the task.
Here are a few common types of AI models:
Decision trees: These are tree-like models that make decisions based on a series of binary splits.
Random forests: These are collections of decision trees that work together to make predictions.
Neural networks: These are a type of model inspired by the structure of the human brain, and they can learn to recognize patterns and features in data.
Support vector machines (SVMs): These are models that find the hyperplane in a high-dimensional space that maximally separates different classes.
K-means clustering: These are models that partition data into a specified number of clusters based on similarity.
Naive Bayes classifiers: These are models that use Bayes' theorem to make predictions based on probabilities.
Logistic regression: These are models that predict the probability of a certain outcome, such as whether a customer will churn.
Gradient boosting: These are models that combine multiple weak learners to create a strong learner.
Once you know what type of AI model you need to solve your problem you have to collect and label a large dataset that is representative of the problem you are trying to solve. For example, if you want to build a system that can identify objects in images, you would need a dataset of images that have been labeled with the names of the objects they contain.
After that you have to preprocess the data by cleaning it and formatting it in a way that is suitable for training a model. This might involve resizing images, normalizing numerical values, or handling missing data.
Then you have to split the dataset into a training set and a test set. The training set is used to train the model, while the test set is used to evaluate the model's performance.
During the training process you will:
Train a model using a machine learning algorithm.
Evaluate the model and fine-tune it as needed.
Repeat the training and evaluation process over and over until the model performs well on the test set.
After training the AI model, you will generally:
Deploy the model and use it to make predictions on new, unseen data.
Monitor the model's performance and fine-tune it as needed to maintain good performance over time.
Retrain the model periodically with new data to ensure that it continues to perform well.
The model learns to make predictions by finding patterns in the training data and must be evaluated on the test set and fine-tune it as needed. This might involve adjusting the model's hyperparameters, adding more training data, or trying a different algorithm. After all that you can deploy the model and use it to make predictions on new, unseen data.

There are many ways to train an AI model, and the best approach depends on the specific problem you are trying to solve and the resources you have available. Some common approaches include:
Supervised learning: This involves training a model on labeled data, where the correct output is provided for each example in the training set. The model makes predictions by learning to map input data to the corresponding output labels.
Unsupervised learning: This involves training a model on unlabeled data, and the model must find patterns and relationships in the data without being told what the correct output should be.
Semi-supervised learning: This involves training a model on a dataset that is partially labeled and partially unlabeled.
Reinforcement learning: This involves training a model to take actions in an environment in order to maximize a reward. The model learns through trial and error, receiving positive or negative feedback based on the actions it takes.
Transfer learning: This involves using a pre-trained model as a starting point and fine-tuning it for a new task, rather than training a model from scratch. This can be useful when there is limited training data available for the new task.
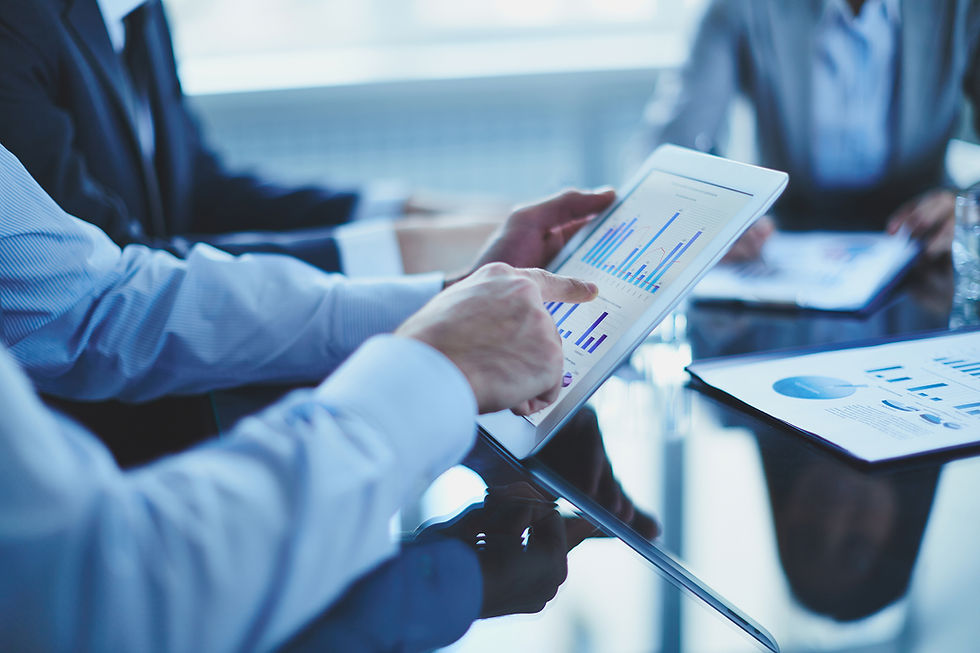
There are other and more specific ways to train an AI model:
Statistical Machine Translation: This method uses statistical models to translate text, and it works by building a translation model based on a large parallel corpus of text that has been translated by humans. The model uses this data to learn the statistical relationships between words and phrases in the source language and their translations in the target language.
Neural Machine Translation: This involves training a model to translate text from one language to another. The model is trained on a large dataset of human-translated sentences and learns to predict the translation of a given sentence in the target language. NMT has significantly improved the quality of machine translation in recent years and has largely replaced older methods such as statistical machine translation.
Generative Adversarial Networks (GANs): These involve training two models, a generator and a discriminator, to work together. The generator produces synthetic data, while the discriminator tries to determine whether each piece of data is real or synthetic. The generator improves over time as it tries to produce data that can fool the discriminator, and the discriminator gets better at identifying synthetic data.
Deep Learning: This involves training a model with many layers of artificial neural networks, which are inspired by the structure of the human brain. Deep learning models can learn to recognize patterns and features in data and make decisions based on that information. Deep learning has been successful in a wide range of applications, including image and speech recognition, natural language processing, and machine translation. It has achieved state-of-the-art results on many tasks and has significantly improved the performance of AI systems in recent years.
Evolutionary Algorithms: These involve training a model by simulating the process of natural evolution, where the fittest individuals are more likely to survive and reproduce. The model is trained by generating a population of potential solutions to a problem and iteratively improving a population of potential solutions through selection and reproduction, and introducing random variations through a process called mutation. Evolutionary algorithms are useful for problems where it is difficult to find an exact solution and can be applied to a wide range of optimization problems.
コメント